Evaluate predictions
When an observation is added to the context from an implicit action and a prediction is made, users should be able to easily evaluate and dismiss it.


When an observation is added to the context of the AI system or a conclusion is reached, I want to evaluate and dismiss it easily, so I can ensure the information is accurate and relevant to my needs.

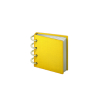
- Transparency in Knowledge Gathering: Make it easy for users to understand the factors that influence and shape the knowledge being gathered. When new information is added to the context, clearly communicate this to the user.
- Control over Assumptions: Provide a simple way for users to dismiss or challenge assumptions to ensure the accuracy and reliability of the knowledge base.

More of the Witlist

Letting people select text to ask follow-up questions provides immediate, context-specific information, enhancing AI interaction and exploration.

Ordering content along different interpretable dimensions, like style or similarity, makes it navigable on x and y axes facilitating exploration and discovery of relationships between the data.
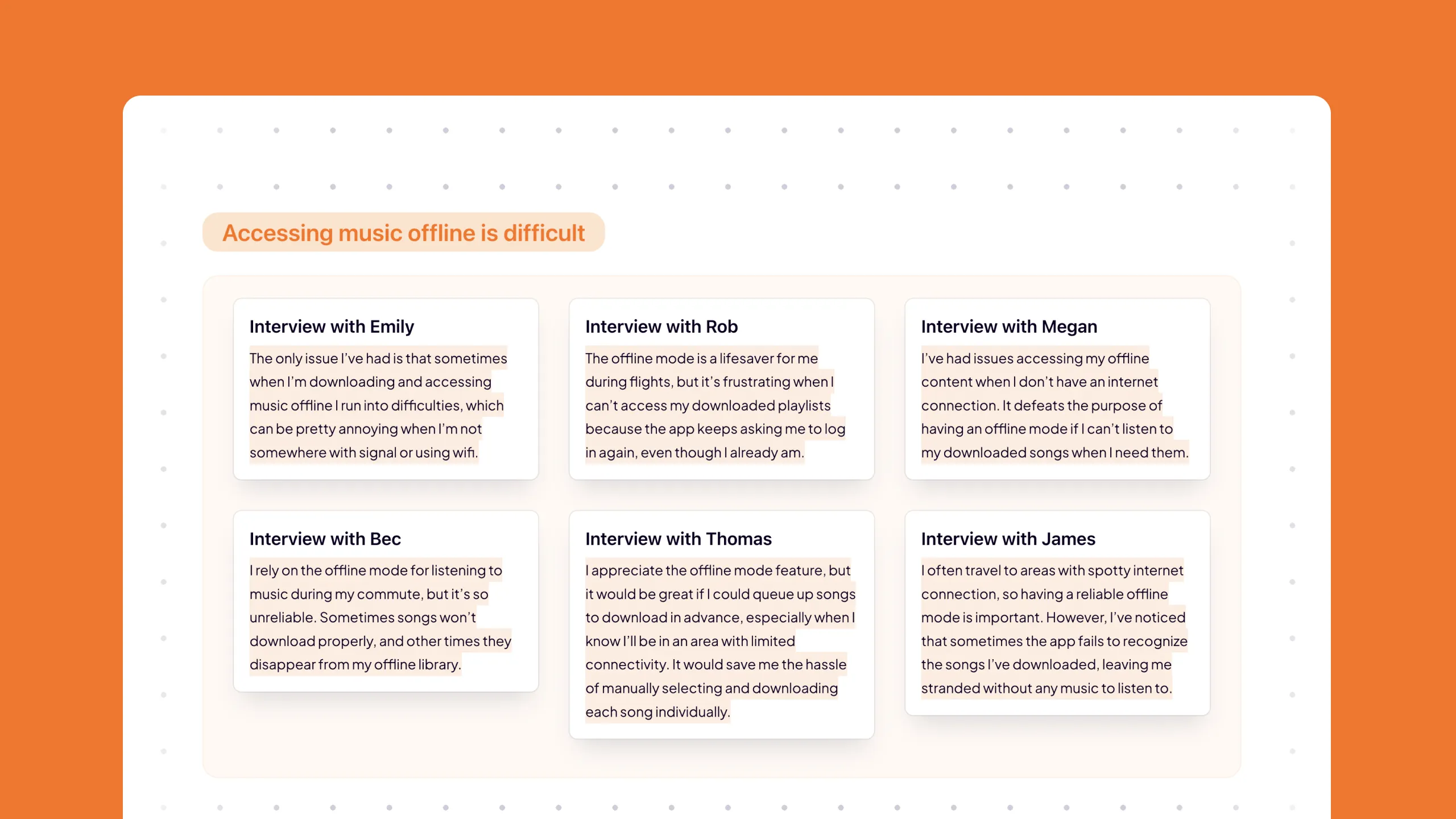
LLM’s are great at organizing narratives and findings. It's helpful to see the sources that support these conclusions, making it easier to understand the analysis and where it comes from.

Embedding models can rank content along virtually any dimension. This capability provides significant value by enabling users to explore and analyze the embeddings to create a spectrum of any features.
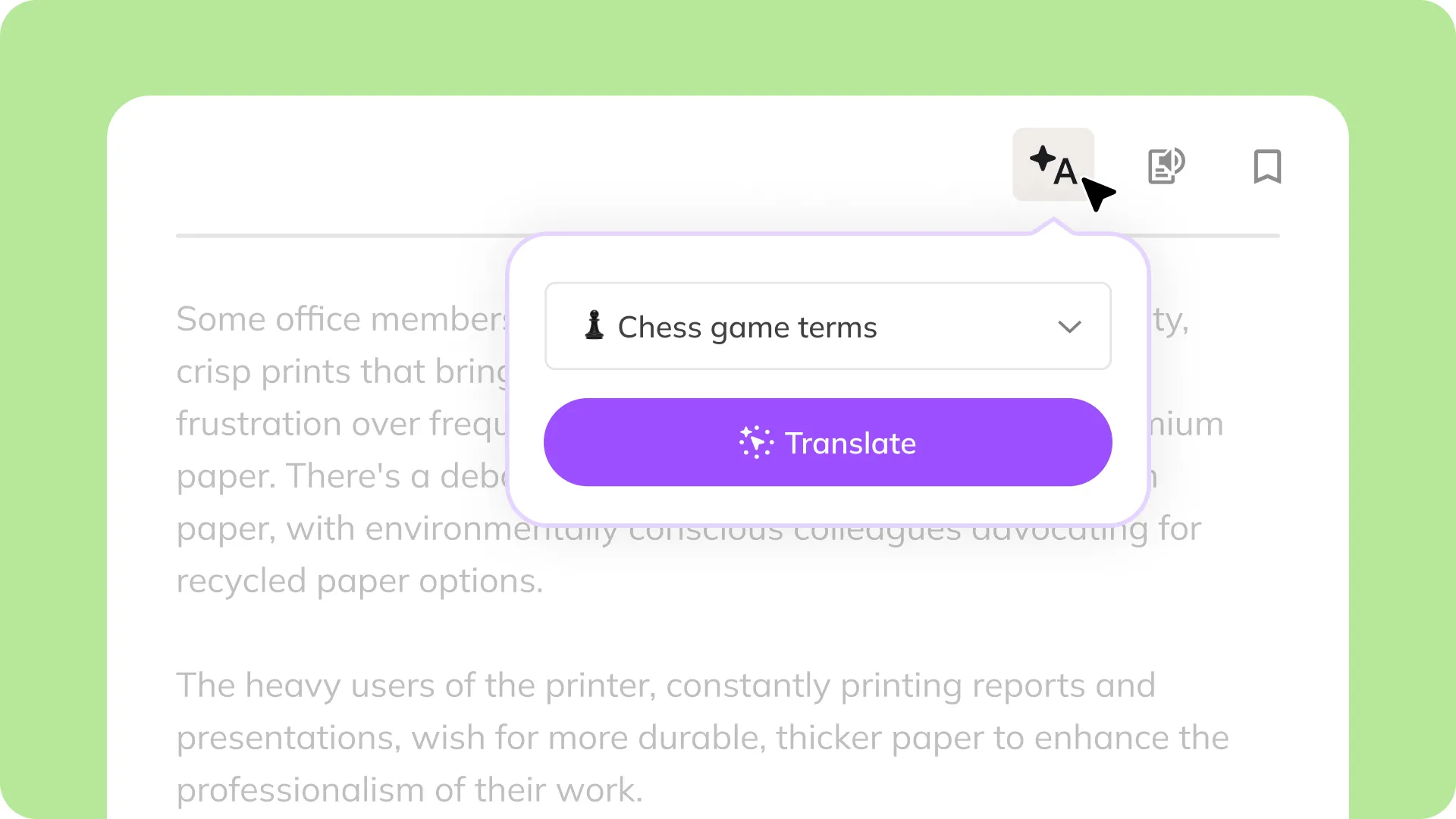
Provide relatable and engaging translations for people with varying levels of expertise, experience and ways of thinking.
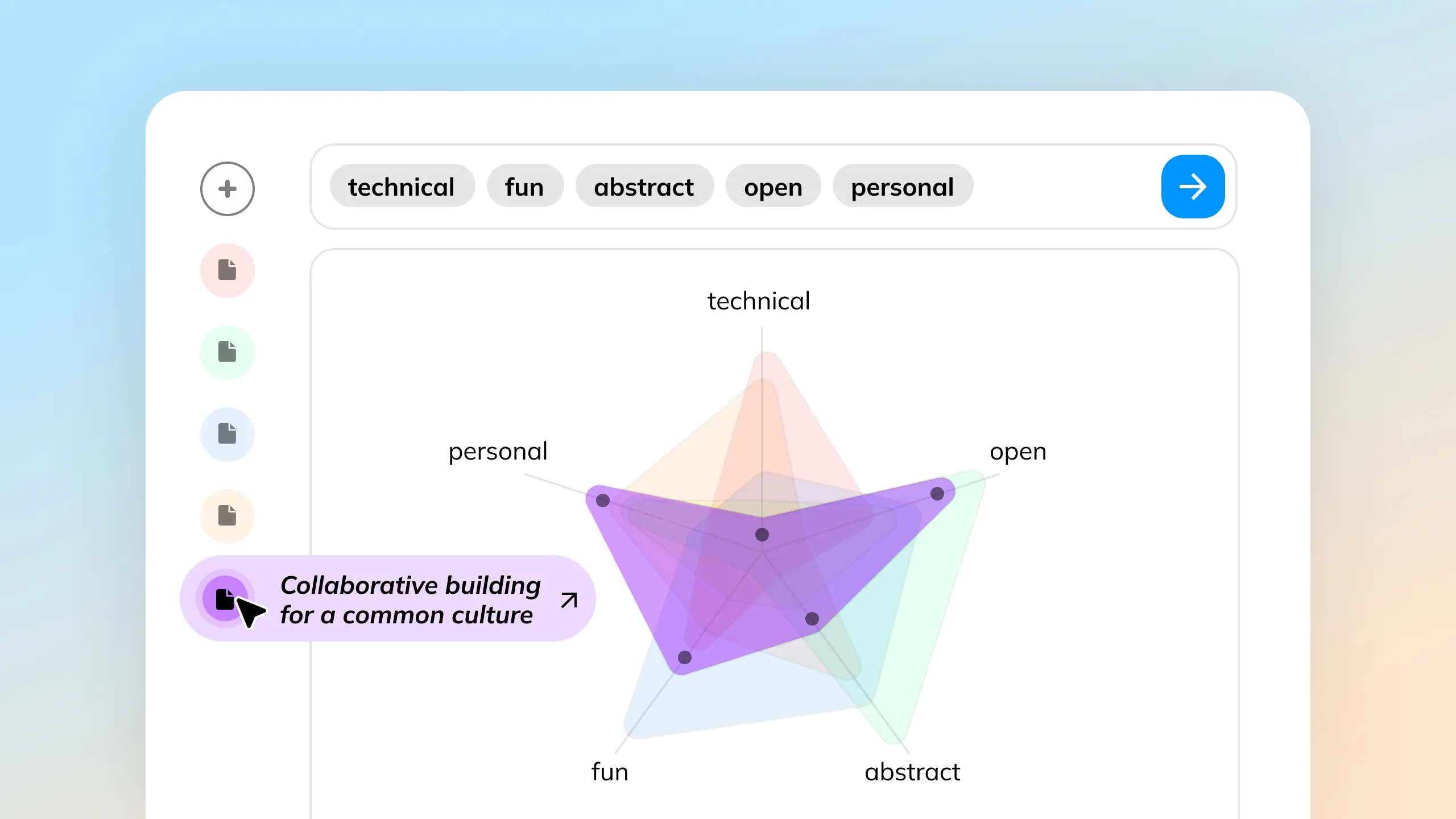
Comprehend and compare large documents by visualizing embeddings and their scores, enabling a clear and concise understanding of vast data sources in a single, intuitive visualization.